Transparency in Copyright and Artificial Intelligence
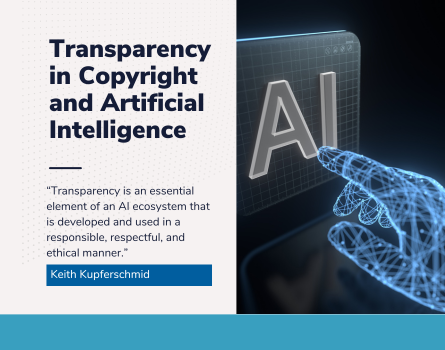
Transparency is an essential element of an AI ecosystem that is developed and used in a responsible, respectful, and ethical manner.
Generative Artificial Intelligence (AI) has raised so many issues for the copyright community. We have blogged about the numerous AI-related copyright infringement cases and the issues that arise in those cases. We have also blogged about copyrightability issues and issues relating to how to draw the line between copyrightable and noncopyrightable works that incorporate AI-generated elements. But one crucial issue that we haven’t blogged extensively about yet is the importance of transparency in AI development.
There are two transparency related issues. One deals with transparency around training data–i.e., AI companies being transparent about what materials are ingested to train their AI systems. The other transparency issue is really more of an output labeling issue as it deals with whether, how, and when to tell the public that something was generated, in whole or in part, using AI. We’ll save the second issue for another blog and instead focus this blog on the first issue.
Why Transparency Is Important
At the time this blog was written we were fast approaching thirty copyright infringement suits brought by copyright owners against generative AI companies (some of which have since been consolidated based on similar claims against the same defendant AI companies). It should come as no surprise that, with all the copyright infringement suits being filed, AI companies have become increasingly secretive and less transparent about the copyrighted works ingested for training their AI systems. For example, Meta refused to disclose the details of how the second version of its LLaMA tool was trained.
Why be so secretive? Well, that’s simple. If a copyright owner discovers that their work has been ingested by an AI developer, it could spur that owner to initiate a copyright infringement suit against the AI developer. Consequently, AI developers have every reason to “hide the ball” so as not to incentivize further litigation.
But as we’ve seen from the many lawsuits that have already been filed, some copyright owners will bring an infringement suit even when they may not have direct evidence that their works have been ingested by an AI developer. In these cases, the copyright owners have demonstrated (or attempted to demonstrate) in their complaints that it is possible to generate AI output which is identical, virtually identical, or at least substantially similar to one or more of their copyrighted works. This establishes, albeit by circumstantial evidence, that the generative AI system could not have produced this output unless it actually ingested and copied the copyrighted works during the training process.
For instance, there are many ongoing lawsuits filed by copyright owners over the unauthorized ingestion of their copyright-protected works to train various AI models that have shown evidence of outputs generated using Large Language Models (LLMs) that include verbatim or near verbatim text of copyrighted literary works. In the New York Times (NYT) v. OpenAI lawsuit, the newspaper publisher sued the AI developer for, among many other things, direct copyright infringement based on copying and use of NYT’s works to train its ChatGPT model. The complaint alleges that NYT’s articles were a prevalent part of datasets OpenAI used to train ChatGPT, and it goes on to show examples of ChatGPT generating verbatim outputs of significant portions of various NYT articles—something it could not possibly do without first ingesting and copying the protected works.
In another case, Concord Music Group v. Anthropic, a group of music publishers accuse an AI developer of violating their copyrights by unlawfully copying and distributing their musical works, including lyrics, to train Anthropic’s Claude chatbot. The complaint alleges that Anthropic scrapes and ingests massive amounts of text (including the plaintiffs’ musical compositions) from the internet and potentially other sources. Importantly, the complaint explains that these materials are not free for the taking and copying simply because they can be accessed online. Showing clear evidence of Anthropic’s copying, the complaint includes multiple examples of near-identical output alongside the copyrighted lyrics to songs by popular artists. As the complaint notes, these examples are not merely substantially similar, but strikingly similar.
Lawsuits have also been filed by visual arts copyright holders that show how AI image generators produce substantially similar versions of their protected works, sometimes even including a copyright owner’s watermark. For example, in Getty Images’ lawsuits against Stability AI in the United States, Getty shows Stability output alongside copyrighted photos to demonstrate clear substantial similarity. As if that wasn’t enough to prove unauthorized copying, the complaint shows how some of the Stability model’s output contains Getty watermarks that the AI developer didn’t even try to remove—which itself could be a violation of the DMCA under section 1202.
While copyright owners in these cases were able to demonstrate copying, copyright owners would likely not need to go through the trouble of showing how closely the AI generated output resembles the copyrighted work in order to prove infringement of the reproduction right.[1] Only the AI developer truly knows (or should know) what is in their training data sets and should be able to provide those details. It therefore is unreasonable to expect copyright owners to shoulder this burden. A transparency disclosure itself would provide the direct evidence copyright owners need to be able to establish copying. In other words, a transparency requirement would make it easier for the copyright owner to prove infringement in court. And, since the last thing any AI developer wants to do is make it easier for a copyright owner to prove them liable for infringement, they have vigorously opposed any transparency requirement.
While it may also be possible to get direct evidence of copying (without the imposition of legal transparency requirements) during the discovery stage of a litigation, that is far from certain. In some cases, a third-party dataset curator, rather than the defendant-AI company may have the information sought. In other cases, the defendant-AI company may not have retained copies or information about what was copied. Even when the information can be obtained through the discovery process, because discovery is an expensive and lengthy process, getting ingestion information through discovery is not the most effective means for getting the information and is a waste of the court’s and the parties’ time. These are all good reasons to have appropriate and effective transparency rules in place.
What this all means in practice is that without appropriate and effective transparency rules, AI developers can exploit copyright owners and creators, especially independent creators, without their knowledge. Of course, there are numerous reasons to impose transparency requirements that have absolutely nothing to do with copyright. For instance, to safeguard against algorithmic biases or ensure that AI models don’t output illegal material like child pornography. But since this is a Copyright Alliance blog, our focus is only on the copyright aspects of transparency.
From a purely copyright perspective, transparency of ingestion of copyrighted works by businesses that offer generative AI systems to the public is essential because it will help ensure that the rights of copyright owners are respected, and that generative AI systems are being developed and implemented in a way that is responsible and ethical. And to the copyright community, that’s what this is all about. The Copyright Alliance and our members are on record as supporting generative AI, so long as it’s accomplished in a manner that is responsible, respectful, and ethical.[2] Transparency is an essential element of each of those benchmarks.
Adequate and appropriate transparency and record-keeping benefit both copyright owners and AI developers in resolving questions regarding infringement, fair use, and compliance with licensing terms. And, of course, transparency has many other benefits unrelated to copyright such as promoting safe, ethical, and unbiased AI systems. Consequently, there can be little doubt that transparency by businesses that offer generative AI systems to the public is a crucial component of any AI policy that will ultimately result in safer and more ethical technologies.
Who and When Should Transparency Apply
AI companies should be required to maintain records of what copyrighted works are being ingested, how those works are being used, and whether the works reside or are stored somewhere within the system or elsewhere. But when it comes to disclosing that information there should be limitations to that requirement. For example, heightened copyright-specific transparency requirements should not be imposed on all AI developers, only those who develop generative AI systems by ingesting material that they do not own, have not licensed, or are not the subject of copyright protection. And those requirements should only be imposed on those entities and individuals that offer generative AI systems to the public.
Importantly, transparency obligations should not apply to works that the AI developer owns or have licensed for ingestion purposes from the copyright owner. Nor should they apply where such obligations would be contrary to or inconsistent with obligations under other laws (such as privacy laws), contracts, or collective bargaining agreements.
For copyrighted works that are owned by the AI developer, that AI developer should not have the same transparency obligations as developers who do not own or have not licensed works ingested for training. The reason being that that there would be no risk of infringement if the AI developer retained all rights that could be implicated by ingesting and copying works as part of training a model.
The licensing exclusion serves several purposes. First, the AI developer should not have any obligations where a work is licensed because if the licensor has concerns with how their work is to be used and protected, those can be addressed in the terms and conditions during the license negotiations. Second, presumably the licensor-copyright owner is compensated for and has authorized the use of their works so there would be no “free-riding” concerns. Third, there really is no reason for the transparency obligations to apply because the licensor-copyright owner is presumably aware that the AI company is ingesting their copyrighted works. And finally, and perhaps most importantly, for those AI companies that oppose transparency, it gives them a simple solution to address their transparency concerns—if they license the work, the transparency obligations would not apply to those works.
Applying Transparency Requirements
Developers of AI models that are made available directly or indirectly to the public that ingest copyrighted works owned by third parties without a license should be required to comply with transparency standards related to the collection, retention, and disclosure of the copyrighted works they use to train AI. Best practices from corporations, research institutions, governments, and other organizations that encourage transparency around AI ingestion already exist, and they enable users of AI systems or those affected by its outputs to know the provenance of those outputs.[3] There is no reason these same responsibilities should not also apply to the ingestion of copyrighted works. However, there is a big difference between voluntary best practices and binding legal requirements, which is why the Copyright Alliance and many of our members are on record as supporting the imposition of legal obligations related to transparency and record keeping.
It is vital that AI developers be legally required to maintain adequate records of what materials were used to train the AI and how those materials were used, and to make those records publicly accessible and searchable, subject to the aforementioned conditions. These records should indicate which copyrighted works are ingested; how those works are used; when the works were ingested; the legal basis for collection of the works; how the work was acquired; whether any modifications, additions, or deletions have been made to a training dataset acquired from a third party; whether copies have been disseminated to third parties; and whether copies of the works are retained. Where copies of the works ingested are retained, records should also indicate how long copies are retained and what security measures are in place to prevent the copies from being leaked through a cyberattack or otherwise or inadvertently disclosed.
With regard to disclosure, copyright owners should be able access transparency records to determine if their works have been trained by that company’s AI model using standard metadata (e.g., ISRC or artist and track name for musical recordings). Where publicly available webpages have been scraped, a searchable database of URLs of those webpages should be made publicly accessible at a centralize location. In addition, each AI model—when prompted—should be required to disclose whether the model was trained on a particular work. Of course, in each of these instances, caution in the manner of disclosure should be exercised so that these public disclosures do not further propagate the spread or use of unlicensed copyrighted works.
None of this should be difficult to do. Nor would it be expensive. The transparency obligations simply require keeping track of what datasets are used when, and if the AI company creates their own datasets from materials that it does not own or has not licensed, what sources they used. While the volume of material used in training is often large, systems are not trained on unorganized raw files. Because the datasets are organized and cleaned before training, a commercial market already exists to help AI developers keep such records.[4] Recordkeeping costs are simply a cost of doing business that is necessary to promote safe, responsible, respectful, ethical, and unbiased AI systems.
In conclusion, if we are going to live in a world with responsible, respectful, and ethical AI then it is essential that strong and effective transparency rules are in place in the United States and abroad that protect the creative community from infringement and misuse of their works.
[1] If the copyright owner also alleges a violation of the derivative work right by virtue of the AI generated output standing alone, the copyright owner would need to show that the allegedly infringing work incorporates copyrightable elements from the copyrighted work. To avoid going down another AI rabbit hole, we’ll leave that issue for a separate blog.
[2] See Copyright Alliance Position Paper on Artificial Intelligence and Copyright Alliance Comments on Artificial Intelligence submitted to the U.S. Copyright Office
[3] See Content Authenticity Initiative, stating that “[o]ur tools make it easy to indicate when AI was used to generate or alter content. Information about specific AI models used and more can be conveyed to viewers, helping to prevent misinformation and increase transparency around the use of AI.”
[4] For example, see Whylabs and Superannotate.
If you aren’t already a member of the Copyright Alliance, you can join today by completing our Individual Creator Members membership form! Members gain access to monthly newsletters, educational webinars, and so much more — all for free!